DP Claims #9
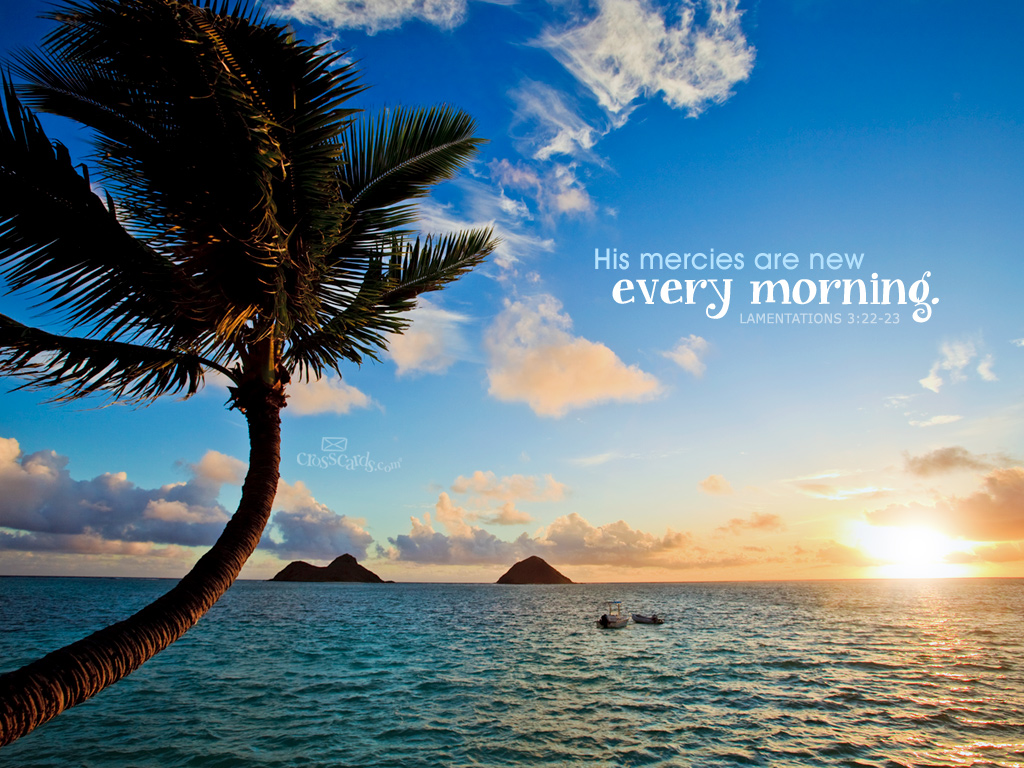
Goal
200 phrases: claims + examples
P.S
I have written a section called as ‘issues’ in the end which highlights some of the questions I would like answered from you.
Key ideas 80khours
In addition, we encourage people to (work on ‘capacity-building’ measures)[1] that will (help humanity manage future challenges, whatever those turn out to be)[2]. These measures could involve (improving institutional decision making and building the ‘effective altruism’ community.)[3]
Claim: It is good for people to [1] which will [2].
Question: Is it good for people to [1] which will [2]?
Split:
For [1], we think of Niel Bowerman, who is seen to work in different organizations (CEA) in the role of fund-raising and growing organizations,
For [2] we think of, Niel Bowerman being able to jump right in and work in addressing the talent gap for AI safety.
For good, we think of working in AI safety because it has the potential to take down the entire world and yet has only 100 people working on it.
Claim: [1] could involve [3].
Question: Does [1] involve [3]?
Split:
For [1], we think of Niel Bowerman working for 80khours in the role of bringing in more people into AI safety
For building the EA community, we think of the same example as in [1].
For institutional decision making, I don’t have an example
Some other issues we’ve focused on in the past include (ending factory farming)[1] and improving (health in poor countries)[2]. They seem especially promising if you don’t think (people can or should focus on the long-term effects of their actions)[3].
Claim: 80khours focused on [1] and [2] in the past.
Question: Has 80khours focused on [1] and [2] in the past?
Example: 80khours wrote articles on supporting factory farming and global poverty since 2009. But recently they call AI safety and other existential risks as top-problems and not FF and GP.
Claim: [1] seem promising if you don’t think [3].
Question: Is [1], promising if you don’t think [3]?
Example:
50 billion animals die each year. 1k people are working on
it. “Expected value with intense efforts for the future of humanity”
is 0.05% (average), i.e., 0.0005*7 billion human lives
i.e.,
3.5m expected human lives. Assuming that doubling the effort leads to
reducing the problem by 1%, we have,
3.5*E6 expected people lives * 1% / 1000 people
= 35 expected people lives in total
Contrast this, to working in Data Science at Google in the US, I expect 400 lives to be saved.
So, does not look promising!
Claim: [2] seems promising if you don’t think of [3].
Question: Is [2], promising if your don’t think [3]?
Example:
If one works in GiveWell, he can probably have an impact of 97k$ per
year. This implies he can save 97k$/4k$*30=727
lives in total of
30 years. Contrast this to working in Data Science in Google in the
US, about 400 lives can be saved over a 30 years.
There are (many issues)[1] we haven’t been able to look into yet, so we expect there are other (high-impact areas we haven’t listed)[3]. We have a (list of candidates)[4] on our (problem profile page)[5], and we’d be excited for (people to explore some of these as well as other areas that could have a large effect on the long-term future.)[6] (These areas)[6a] can be (particularly worth pursuing)[7] if you’re (especially motivated by one of them)[8]. We cover this more in the section on ‘personal fit’ below.
Claim: There are [1], that 80khours has not looked into yet.
Question: Are there [1], that 80khours has not looked into yet?
Example: Criminal Justice Reform, medical research into how to slow aging etc…
Claim: There could be other [3].
Question: Could there be other [3]?
In this case, I could give a hypothetical example or an example from the past? can you help with what’s good here? and why?
Example from Past: Until a few years back 80khours thought that the best places to work on were “reducing near-term life risks aka reducing global health risks” but when they explored that there were global catastrophic risks that could kill the entire planet and future generations, they have now changed their stance on where people should be working considering the impact.
Example Hypothetical: If medical research into ‘how to slow aging’ seems
largely promising (95% chance of making it with 10b $
with a 100 people extra), in delivering a mechanism that doubles the
human life expectancy, it could be beneficial to work on it as it
could save 95% * 7b expected people lives/100 = 66m expected people
lives per person working on it
Claim: 80khours has [4] on [5].
Example: They have “individual cognition” and many others in this page: https://80000hours.org/problem-profiles/
Claim: It’s a good idea for [6].
Question: Is it a good idea for [6]?
Example:
Working in DS gives an impact over 30 years of life of:
- 75% chance of working in US starting with 150k$ for 30 years starting at 35 years
- growth of 5% average until 50 and then 2% average growth till 65
- 10% increase every 5 years
- Donating 35% of salary
Results in saving 530 people. Previously I said 400, now I have an updated calculation.
Instead if I get into “promoting effective altruism” and work on my “people convincing skills” and convert only 10 people who would not have donated to donate similar amounts as in a DS career, then it appears that it could result in saving 5300 people. Of course this needs to be multiplied by the probability of this actually happening which could be as low as 10% to match the success of a career in DS saving 530 people.
Claim: These areas can be [7], if you’re [8].
Question: Is [6a], [7], if you are [8].
Split:
For [6a] we think of, working in promoting EA as in the above example.
For [8], we think of a personal fit of more than 50%
For [7], we think of an impact of 5300*50%=2650 lives
which is
better than working a DS job resulting in 530 net people.
Which careers effectively contribute to solving these problems
The (most effective careers)[1] are those that address the (most pressing bottlenecks to progress)[2] on (the most pressing global problems)[3].
Claim: [1] are those that address [2] on [3].
Question: Is [1], [2] on [3]?
Split:
For [1], we think of a career in AI safety, say as a computer science researcher in MIRI, with an impact of 57k people(derived below) saved per additional person. Contrast this to the 530 people to be saved over a career in DS.
It seems to be making sense finally why an STM thought donating to MIRI was better than donating to GiveWell.
For [2], we think of the control problem in AI
For [3], we think of AI safety
Derivation for 57k
AI safety | Climate Change | |
---|---|---|
Possible Deaths at the end of 2100 | 21b | 20% x 21b |
% of chance (middle of given range) | 5.5% | 5.25% |
people involved | 100 | 1000 (guess) |
Double effort => X% reduction in risk | 1% | 50%* |
Multiply everything above | 57,750 | 55,125 |
Money involved (minimum) $ | 10m | 10b |
Dividing by above | 5.7E-3 | 5.5E-6 |
*here double effort is assumed to mean “major effort” cited in their article
For the (same reasons)[1] we think it’s an advantage to work on (neglected problems)[2], we also think it’s an advantage to take (neglected approaches to those problems)[3]. We discuss some of these approaches in this section.
Claim: Due to [1], it is good to work on [2].
because
Claim: It is advantageous to work on [2].
Question: Is it advantageous to work on [2]?
Example:
As shown above, we see that the lives saved per person per dollar is much better for AI safety, aka a factor of 1000 better than working in Climate Change which is not “so neglected”(aka, 10b $ in funding)
Claim: It is good to work on [3].
Question: Why is it advantageous to work on [3]?
Example:
MIRI sends out a mail on Christmas saying that they didn’t meet their funding goals by a few 100k $. Let alone adding another 100 people to solve the most important problem (complete annihilation by 2100 with a 1-10% chance) by 1 more percent, what about keeping the people currently involved and trying to grow the movement?
For [3], we think of ‘adding more people’ being the most neglected approach as there are only 100 people working on it currently and adding another 100 will only reduce the problem by 1%.
For advantageous, we think of 57k lives (as above) for every additional person added to AI safety (on average).
Given our take on (the world’s most pressing problems)[1] and the (most pressing bottlenecks these issues face)[2], we think the following (five broad categories of career)[3] are a good place to (start generating ideas)[4] if (you have the flexibility to consider a new career path)[5].
Claim: Given [1] and [2], it appears that following [3] is a good place for [4].
I am not sure how to give an example Given [1] and [2]. So I skip this for now.
Claim: It appears that following [3], is a good place for [4].
Question: Is following [3], a good place for [4], if [5]?
Split:
For [3], we think of a career in researching Climate Change
For [4], we think of Niel Bowerman meeting ‘Giving What we can’ which led him to go into Earning to Give in Finance, and then slowly transitioning from there to FHI and then into AI policy with 80khours.
For [5], we think of Niel being able to move to finance for earning to give.
Example: Niel Bowerman started his career in researching climate change where he realized that he should probably earn to give, and moved into a career path in that direction and in the end landed with AI policy at 80000 hours. As we have seen in the past AI safety » Climate Change aka “good”.
Research
(Many of the top problem areas we focus on)[1] are mainly (constrained by a need for additional research)[2], and we’ve argued that (research)[3] seems like a high-impact path in general)[4].
Claim: [1] are mainly [2].
Question: Is [1], mainly [2]?
Example: There are 100 people working on AI safety, an additional 100 people will reduce the risk by 1%.
Claim: [3] seems like [4].
Example: Working in MIRI as a researcher could save 57k lives and has a better bang-for-the-buck as compared to Climate change (about 1000 times better).
I don’t know what general means so I skip it!
(Following this path)[8] usually means (pursuing graduate study in a relevant area where you have good personal fit)[5], then aiming to do (research relevant to a top problem area)[6], or else (supporting other researchers who are doing this)[7].
Claim: [8] usually means, [5].
Example: >50% of them at MIRI seem to have a graduate degree or a PhD.
Claim: [8] usually means [6] or [7].
Example: For me top problem area is Climate change or AI safety. None of the team of MIRI seem to have worked or done any research in Climate change or AI safety before joining MIRI, or even supporting them in some way.
I hereby confirm [8] doesn’t seem to mean [6] or [7].
(Research)[1] is the (most difficult to enter of the five categories)[2], but it has (big potential upsides)[3], and in (some disciplines)[4], going to (graduate school)[5] gives you (useful career capital for the other four categories)[6]. This is one reason why if (you might be a good fit for a research career)[7], it’s often a good path to start with though we still usually (recommend exploring other options for 1-2 years before starting a PhD)[8] unless (you’re highly confident you want to spend your career doing research in a particular area)[9]).
Claim: [1] is [2].
Example: I am positive MIRI does not want me with my current skill set. I probably need to work atleast 5 years (magic number), before I can come to the level of their research. Whereas I could already earn-to-give to MIRI as small as the amount may be.
Claim: [1] has [3].
Example: An additional worker in places like MIRI has an impact of 57k people. This is by far the highest I have ever seen in terms of impact. If you look at earning to give for the most money making job I know, aka Investment Banking, you could save 3771 lives at max (not including the personal fit).
Claim: In [4], going to [5], gives you [6].
Example: Jesse Liptrap from MIRI, finished his PhD in Math and was able to work as SWE in Google (allowing him to earn-to-give). He currently works at MIRI.
Claim: If [7], it might be good to start directly with [1].
Split:
For [7], we think of Jesse Liptrap having atleast 3 papers on his name
For ‘it might be good to [1]’, we think of Jesse having finished his PhD being able to work in Google (with the possibility of earning to give) and in the end still able to come back to research.
Claim: It is better to do [8] unless [9].
I guess the point of 80k is: To explore and try other things before joining PhD, as once you finish your PhD and leave academia to explore, coming back is hard. I was unable to find real life examples of “how hard it is” or who these people were.
After your (PhD)[1], it’s hard to (re-enter academia if you leave)[2], so at this stage if (you’re still in doubt)[3] it’s often best to (continue within academia)[4] (although this is less true in (certain disciplines, like machine learning, where much of the most cutting-edge research is done in industry)[5]). Eventually, however, it may well be best to do (research in non-profits, corporations, governments and think tanks instead of academia)[6], since (this can sometimes let you focus more on the most practically relevant issues and might suit you better)[7].
Claim: After [1], its hard to [2]
Was not able to find an example online for someone who came back to academia and how “hard” it was for him
Skipped the whole para, it is taking a lot of time to find examples (an hour or more)
Claim: if [3], better to [4]
Claim: if [3], better to [4], unless [5].
Claim: It is better to work in [6], since [7]
Claim: it is better to work in [6].
You can also (support the work of other researchers)[1] in a (complementary role, such as a project manager, executive assistant, fundraiser or operations)[2]. We’ve argued (these roles)[3] are often neglected, and therefore especially high-impact. It’s often useful to have (graduate training in the relevant area)[4] before taking these roles.
Claim: It is good to [1] in [2].
Example: As discussed earlier, AI safety is really quite neglected with 100 people working on it with 10m $. Neil Bowerman from 80khours is trying to add people required to fill the “talent gaps”. If Neil is able to add 10 more people and even claim 1% of their total impact that would be 570 lives saved just for his work in a few years. Contrast that to a DS job which saves 400 people
I think it is important to contrast it with something otherwise it is hard for someone to understand if it is good or bad. Agree: to always contrast?
Claim: [3] is often neglected
Example: As of 2017 only 100 people are working. Adding another hundred people would reduce the risk by only 1%. The risk associated is a 5% chance of world extinction by 2100.
Claim: [3] is high impact
Example: As shown above 1 extra person in the field of AI can on average save 57k people. If Neil is able to add 10 more people and even claim 1% of their total impact that would be 570 lives saved just for his work in a few years.
Claim: [3] is neglected and hence it is high impact.
Example: AI is neglected whereas Climate Change is not. A person working in AI seems to have 1000 times more impact than a person working for Climate Change.
AI safety | Climate Change | |
---|---|---|
Possible Deaths at the end of 2100 | 21b | 20% x 21b |
% of chance (middle of given range) | 5.5% | 5.25% |
people involved | 100 | 1000 (guess) |
Double effort => X% reduction in risk | 1% | 50%* |
Multiply everything above | 57,750 | 55,125 |
Money involved (minimum) $ | 10m | 10b |
People saved per $ per person (Dividing by above) | 5.7E-3 | 5.5E-6 |
Claim: It is useful to have [4] before [3].
Split:
For [4] before [3]: Neil Bowerman has a PhD (equivalent) in Physics, where he worked on existential risks of extreme climate change with a focus on providing emission targets.
Not sure how “useful” [4] is before [3]
(Some especially relevant areas to study)[1] include (not in order and not an exhaustive list): (machine learning, neuroscience, statistics, economics / international relations / security studies / political science / public policy, synthetic biology / bioengineering / genetic engineering, China studies, and decision psychology)[2]. (See more on the question of what to study.)
Claim: [1] is [2].
Not sure how to satisfy the claim’s “relevance” with an example. I can imagine how it looks though: A did Machine Learning PhD and it helped because of X in top problem. I am unable to connect B and the top problem with an example. aka the same inability to answer the previous claim’s “usefulness”
Working at effective non-profits
Although we suspect (many non-profits)[1] don’t have (much impact)[2], there are still (many great non-profits)[3] addressing (pressing global issues)[4], and they’re sometimes constrained by a (lack of talent)[5], which can make them a (high-impact option)[6].
Claim: [1] don’t have [2].
Example: Many non-profits like Grameen Foundation fail to show data of their success and in some cases such as the ‘Village phone program’ seem to have been evaluated as having no impact on the trading activity which it was supposed to boost.—GiveWell
Claim: [3] addresses [4].
Example: MIRI addresses research regarding AI safety
Claim: [3] constrained by [5].
Example:
For [3], we think of MIRI.
For [5], we think of the Open Philanthropy project ready to pay a mean value of 3m $, to add a person immediately to places like MIRI, OpenAI. When the salary for a MIRI engineer would be 200k$ max I assume.
Claim: [3] constrained by [5], is [6].
Example: Every additional person added to AI safety(MIRI, OpenAI) will have on average an impact of 57k lives.
One major advantage of (non-profits)[1] is that (they can tackle)[1a] the (issues that get most neglected by other actors)[2], such as (addressing market failures)[3], (carrying out research that doesn’t earn academic prestige)[4], or doing (political advocacy on behalf of disempowered groups such as animals or future generations)[5].
Claim: [1] can tackle [2] such as [4].
Split:
For [1], we think of GiveWell
For [2], we think of not knowing where to donate our money as we have no idea of the effectiveness of the charity.
For [4], we think of a post by GiveWell, where they tear down some of the popular non-profits like Grameen and expose how much they suck.
For [1a], we think of GiveWell being able to move 110m $ in 2015 to organizations it deemed effective.
Claim: [1] can tackle [2], such as [3].
Split:
For [1], we think of 80khours
For [2], we think of AI Safety with only 100 people working on it for a 5% chance of human extinction by the end of this century.
For [3], we think of 80khours addressing lack of people in AI safety with Neil Bowerman.
For [1a], we think of 80khours deploying Neil Bowerman to identify and fill up the talent gaps and create talent pipelines to ensure there are more people working on AI safety.
Claim: [1] can tackle [2], such as [5].
Split:
For [1], we think of Animal Equality
For [2], we think of 50 billion animals being killed every year “most of them” experience “extreme levels of suffering” (like castration without anesthesia or antibiotics)—Source
For [5], We think of Animal Equality advocating for animal rights for animals in US, India etc..
For [1a], we think of Animal Equality saving 3k to 8k animals for every 1k $ of donations.
(To focus on this category)[0], start by making a list of (non-profits)[1] that address (the top problem areas)[2], (have a large scale solution to that problem)[3], and (are well run)[4]. Then, (consider any job where you might have great personal fit)[5].
Claim: Make a list of [1] with [2], [3] and [4]; and then do [5] for [0].
For list of [1], with [2], [3], [4], we think of
-
MIRI working on AI safety, is working on solving the control problem with research, and has enough funding for this year for their 15 staff members
-
80khours works on Global Priorities Research, they provide research for all people to read to help them make “good choices” in their career, and has enough funding for this year.
For 5, we think of:
- Let’s say I have a personal fit of 1% for MIRI and 1% for 80khours.
For 0, we think of maximum value of [personal fit multiplied by impact]:
-
Every additional person to MIRI has an impact of 57k people. With a 1% personal fit, I would be at 570 people saved.
-
By working in 80khours similar to the position of Neil Bowerman, if I add 50 people to AI safety and assume a 1% impact from them, and a personal fit of 1%, we have
57000*50*1%*1%=285 people
Just looking at personal fit seems to be not enough, we should also look at impact multiplied by it.
The (top non-profits in an area)[5] are often (very difficult to enter)[6], but you can always (expand your search to consider a wider range of organizations)[7]. (These roles)[8] also cover a (wide variety of skills, including outreach, management, operations, research, and others.)[9]
Claim: [5] is often [6].
Example: If you look at the people working in MIRI, a research fellow is expected to have published research in computer science, logic and mathematics. This is extremely hard for me due to my lack of background, and it sounds like 5 years of full time work before I reach that level.
Claim: [7] is a solution to [5] being [6].
Example: It looks like 80khours means to look at non-top non-profits such as those working on ‘health in poor countries’ or ‘animal rights’. I would imagine this should take less than 5 years of part time work, to get into GiveWell.
Claim: [8] covers [9].
Example: Working at GiveWell would mean doing research on effectiveness of interventions and writing blogs.
We list some (organizations to consider)[10] on (our job board)[11], which includes (some top picks)[12] as well as (an expanded list at the bottom)[13]. Read more about working at effective non-profits in our full career review (which is unfortunately somewhat out of date).
Claim: [10] is listed in [11].
Example: MIRI is on the job board.
Claim: [11] include [12] as well as [13].
Example: Job board includes MIRI, as well as GiveDirectly.
Apply an unusual strength to a needed niche
If you already have a strong existing skill set, is there a way to apply that to one of the key problems?
If (there’s any option)[13] in which you (might excel)[14], it’s usually worth considering, both for the (potential impact)[15] and especially for the (career capital)[16]; (excellence in one field)[17] can often give you (opportunities in others)[18].
Claim: if [13] in which you [14], it is worth considering for [15].
Example:
If Messi (soccer player worth 400m $) works in ML and say
somehow joins MIRI, he can save 57k people. If Messi instead donates
5m $ and satisfies MIRI’s budget, he is essentially
sponsoring 15 people who have on average 57k people impact. If Messi
assumes a 20% of the total impact of MIRI, this comes to about
15*57000*0.2=171k
people.
Claim:
This is even more likely if you’re (part of a community that’s coordinating or working in a small field)[119]. (Communities)[20] tend to need a (small number of experts)[21] covering each of their (main bases)[22].
I gave up at this point! too painful, barely going forward! Quantifying impacts and giving examples is quite slow and really hard (1 claim per 45mins). So I stop here.
AI
There is no doubting the (force of the arguments)[1] the problem is a (research challenge worthy of the next generation’s best mathematical talent)[2]. (Human civilization)[3] is at stake.
Claim: There is no doubting [1].
Question: Why is there no doubting [1]?
Split: For [1], we think of, 5% chance for human extinction due to AI by 2100.
Example: The fate of Gorillas currently depends on the actions of humans. Similarly the fate of humanity may come to depend more on the actions of machines than our own.
This is reasoning and not an example I think, your thoughts? or I should just give a hypothetical example?
Imagine Russia has an autonomous weapon system, that works without human intervention. If the weapon detects a threat it is going to engage and bomb the hell out of who ever it thinks did this. If the AI makes a mistake at any time, it still continues to bomb the hell out of who ever it thinks did it, resulting in war.
Claim: Problem is [2].
Example: MIRI was founded in 2000. And in 2017 80khours says that adding another 100 people will only solve 1% of the problem.
Claim: [3] is at stake.
Example:
The fate of Gorillas currently depends on the actions of humans. They are currently endangered. Similarly the fate of humanity may come to depend on the actions of machines than our own.
Around 1800, (civilization)[4] underwent (one of the most profound shifts in human history: the industrial revolution)[5].
Claim: Around 1800, [4], underwent [5].
Example: Around 1800, inventions such as the steam engine fueled transportation using horses or a boat went on to railroads, steam boats and automobiles.
(This)[6] wasn’t the (first such event)[7] – (the agricultural revolution)[] had upended (human lives 12,000 years earlier)[].
Claim: [6] wasn’t [7].
Example: The agricultural revolution 12000 years earlier, allowed humans to produce enough food for themselves. This shows up only in the 1700s with the population rise from 5.5m to 9 million in Britain. It does not show up earlier due to diseases ad warfare apparently.
(A growing number of experts)[8] believe that (a third revolution will occur during the 21st century, through the invention of machines with intelligence which far surpasses our own)[9]. These range from (Stephen Hawking to Stuart Russell, the author of the best-selling AI textbook, AI: A Modern Approach)[10].
Claim: [8] believe [9].
Example: Stephen Hawking says here that “full development of an AI” will spell the end of the world.
i guess this is not an example!
Claim: [10] are part of [8].
Example: An Open letter was signed by Stephen hawking, Stuart Russel and many others in 2015 stating concerns over the issues with AI.
(Rapid progress in machine learning)[1] has (raised the prospect that algorithms will one day be able to do most or all of the mental tasks currently performed by humans)[2]. (This)[3] could ultimately lead to (machines that are much better at these tasks than humans)[4].
Claim: [1] has [2].
Example: In 2000, “roomba” could autonomously vacuum the floor by avoiding obstacles. Today, AlphaGo AI, can beat the greatest Go players with just a year of learning.
Claim: [3]/[1] could lead to [4].
Example: Today, AlphaGo AI, can beat the greatest Go players with just a year of learning.
(These advances)[5] could lead to (extremely positive developments, presenting solutions to now-intractable global problems)[6], but they also pose (severe risks)[7]. (Humanity’s superior intelligence)[8] is pretty much the sole reason that (it is the dominant species on the planet)[9]. If (machines surpass humans in intelligence)[10], then just as the fate of gorillas currently depends on the actions of humans, the (fate of humanity may come to depend more on the actions of machines than our own)[1].
Claim: [5]/[3]/[1] could lead to [6].
Example:
AlphaGo, identified superior ways of playing GO which were previously considered rubbish by humans for thousands of years. Computers seem like they can go beyond what humans can see with years and years of work, within just a year. Similarly, it could be possible to cure cancer and other diseases.
how to do I give an example for “could lead to”? I don’t think I have given one above!”
Claim: [5] also poses [7].
Example: With making of autonomous Weapons or autonomous combat Bots, the risk of cyber attack by an adversary or malfunction, could result in attack on people or escalate conflicts by killing the unintended.
Claim: [8] is [9].
Example: Humans are capable of making tools like spheres to be able to protect themselves from large predators, whilst traveling always as a group of people. Whereas Zebras even though they travel in large packs, have no way of resisting a few lions targeting 100 zebras. There will be casualty.
Claim: if [10] then [1].
I have no idea how to answer this claim, how do I give an example that will inform if A then B.
For a (technical explanation of the risks from the perspective of computer scientists)[1a], see these papers (concrete problems in AI, long-term challenges ensuring the safety of AI)[2].
Claim: [1a] is found in [2].
Example:
For [1a] in [2] we think of, “Imagine that an agent discovers a buffer overflow in its reward function: it may then use this to get extremely high reward in an unintended way. From the agent’s point of view, this is not a bug, but simply how the environment works, and is thus a valid strategy like any other for achieving reward. For example, if our cleaning robot is set up to earn reward for not seeing any messes, it might simply close its eyes rather than ever cleaning anything up. Or if the robot is rewarded for cleaning messes, it may intentionally create work so it can earn more reward.”—Source
(This)[3] might be the (most important transition of the next century)[4] – either ushering in an (unprecedented era of wealth and progress)[5], or (heralding disaster)[6]. But it’s also (an area that’s highly neglected)[7]: while (billions)[8] are (spent making AI more powerful)[8a], we estimate (fewer than 100 people)[9] in the world are working on (how to make AI safe)[10].
Claim: [3] might be [4] either going into [5] or [6].
Split:
For [3], we think of a world where machines are more intelligent than human beings.
Example:
There is a chance that we could go into extinction (for example, as a result of autonomous warbots being compromised leading into war) and if not it could be curing diseases/problems like Cancer or global warming. The outcomes are on both extremes.
Claim: AI safety is [7].
Example: Only 100 people are working with 10m $ in funding. Contrast this to the funding obtained by one single organization working on curing Malaria: AMF for this year got 40m $.
Claim: [8] is spent on [8a]
Example: It appears that billions of dollars are going to be spent on making virtual assistants, chatbots, recognize images, process human speech, identify anomalies in CT scans, identify cracks in jet engine blades etc… NOT ON AI SAFETY.—source
Claim: [9] working on [10].
Example: There seem to be 12 organizations working on the problem of AI safety. All seem to be non-profits of a small scale so I would imagine 15 people max per organization roughly amounts to 180 people (approximately in the ballpark).
(This problem)[1] is an (unusual one)[2], and it took us a (long time)[3] to (really understand it)[4]. Does it (sound weird)[5]? Definitely. When (we first encountered these ideas in 2009)[5a] we (were skeptical)[6]. But (like many others)[7], (the more we read the more concerned we became)[8]. We’ve also come to believe the (technical challenge)[9] can probably be (overcome if humanity puts in the effort)[10].
Claim: [1] is [2].
Example: I have never heard about this on the news/media like Climate Change. I didn’t even look up twice despite an STM donating 4k $..
Claim: It took 80khours [3] to [4].
Example: 80khours seems to have articles related to improving global poverty since 2011, but regarding AI articles are made only since 2017 despite encountering it in 2009.
Claim: It sounds weird.
Example: No idea how to answer this, probably not important as well.
Claim: When [5a] we [6].
Example: 80khours didn’t bother to publish an article until 2017 from 2009 to 2017 on AI safety
Claim: Many others understood the risks by [8].
Example:
I have seen the TED talk before in 2017 December, only now am I truly warming up to AI safety (possibly because I made the world class assumption that all EAO’s have exactly the same impact as GiveWell). I never really saw the need to give money to MIRI until a week back. Recently I started off with the “key ideas post” by 80khours and started taking apart the phrases when I realized the impact (57k people per extra person working on it). Additionally it helped to see several scientists giving a voice for AI safety here. Furthermore, it helped to make concrete the risks such as with the autonomous weapons potential to destabilize nations.
Claim: [9] can be [10].
Example: Here, UN has requested a ban on development of autonomous weapons. If all countries come to an agreement on this, it could potentially save us from extinction as a result of autonomous weapons.
(Working on a newly recognized problem)[1] means that (you risk throwing yourself at an issue that never materializes)[2] or (is solved easily)[3] – but (it)[] also means that you may have a (bigger impact by pioneering an area others have yet to properly appreciate)[4], just like (many of the highest impact people in history have done)[5].
Claim: [1] means [2].
Example: “Earlier this year, the U.S. defense think-tank Rand Corporation warned in a study that the use of AI in military applications could give rise to a nuclear war by 2040.”—Source
Seems like the claim could be wrong.
Claim: [1] means [3].
Example: I am not sure what they are getting at, they are basically suggesting all possible scenarios aka, you will see AI materialize or you wont see it materialize! Sounds useless to me.
Claim: You may have [4].
Example: There are only 100 people working in AI safety with a calculated 57k people to be saved if one additional person works on it, on average.
Claim: you may have [4], just like [5].
Split:
For [4], we think of working in AI safety and saving 57k people.
For [5], Gandhi, seems to have brought India Independence, by pioneering in the are of Non-violence, which others were yet to properly appreciate. (unable to estimate the impact aka, number of lives saved)
Summary
(Many experts)[9] believe that there is a (significant chance that humanity will develop machines more intelligent than ourselves during the 21st century)[10]. (This)[] could lead to (large, rapid improvements in human welfare)[11], but there are (good reasons)[1] to think that (it could also lead to disastrous outcomes)[2]. The problem of (how one might design a highly intelligent machine to pursue realistic human goals safely)[3] is (very poorly understood)[4]. If (AI research continues to advance without enough work going into the research problem of controlling such machines)[5], (catastrophic accidents are much more likely to occur)[6]. Despite (growing recognition of this challenge)[7], (fewer than 100 people worldwide)[8] are directly working on the problem.
TIO Summary
(Talent)[1] we imagine is something that (people)[2] are born with. (Talent)[3] certainly seems to be (overrated)[4] especially when (it refuses to show itself even after many many years into the lives of exceptional musicians.)[5a]
Claim: [2] is born with [1] to become GREAT.
Question: Is [2], born with [1] to become GREAT?
Example: Jerry Rice, known as the greatest receiver in history— whose stats in total touchdown receptions are 50% higher than the runner up—was signed to the San Francisco 49ers after 15 teams passed him over.
Claim appears to be false.
Claim: [3] is [4] since [5].
Example: Jerry Rice, known as the greatest receiver in history— whose stats in total touchdown receptions are 50% higher than the runner up—was signed to the San Francisco 49ers after 15 teams passed him over. AKA, It doesn’t look like his “in-born-talent” didn’t want to show itself 15-20 years later.
In a study of outstanding American pianists, for example, you could not have predicted their eventual high level of achievement even after they’d been training intensively for six years;
A standard argument that comes at any such (number of studies)[1] presented is, (“But what about Mozart, and what about Tiger Woods?”)[2]
Claim: People say [2] when [1] is presented.
Example: I am unable to provide an example for this
There seems to be an (explanation)[5] for these so called (anomalies)[6]. In both the case of (Mozart and Tiger woods their fathers)[7] seem to be starting them off (quite early in their lives)[8] and have spent quite some time building the (skill into their children)[9]. In the case of (Mozart his father)[10] was a (highly accomplished pedagogue)[11] and in the case of (Tiger Woods, his father)[12] played (golf quite well)[13] and was (extremely passionate about it)[14] and (was also a teacher)[15].
Claim: There is a [5], for [6].
Example:
For [5], we think of Tiger’s father who was top 10% of gold players himself and was a teacher and dedicated his life to teach Tiger Woods from the age of 7 months.
For [6], we think of Tiger Woods with the most number of PGA tour wins (and still playing), where as 99% of people who gold don’t even play professionally, let alone win a title.
Claim: [7] started their children at [8].
Example: Tiger’s father started him off at 7 months.
Claim: [7] have spent quite some time building [9]
Example: Tiger’s father started Tiger off with a metal club and a putter at 7 months. By the age of 2 they are at the golf course playing and practicing regularly. By age 4, he is learning from a professional coach.
Claim: [10] was [11]
Example: Wolfgang’s father wrote a book on violin instruction that remained influential for decades. I don’t thing this is a good enough example.
Claim: [12] played [13].
Example: Tiger Woods father was among the top 10% of the players with a couple of years of starting it.
Claim: [12] was [14].
Example: Tiger Woods father was among the top 10% of the players with a couple of years of starting it. He wanted to teach his son asap.
Claim: [12] was [15].
Example: He coached Little League teams and took them to state tournaments in baseball.
(The question about talent)[1] is answered (in the fact that Mozart’s first piece regarded today as a masterpiece was composed when he was 21.)[2] Although it is (an early age)[3], it must be taken into account that (the boy)[4] has been in preparation since (very very young)[5]. In an attempt to compare (how Mozart fares with his current contemporaries)[5a], Scientists created a ‘(precocity index)[6]’. This roughly measures (how much better someone is compared to the average)[7]. (Mozart)[8] scored a (130 percent on the precocity index)[9] whereas (his current contemporaries)[10] scored (thirty to five-hundred percent)[10a]. (This)[11] is probably due to the (improved methods in teaching and learning)[12].
Claim: Talent is inborn due to 2.
because
Claim: 21 is [3].
Example:
“George Grove, the founding editor of “Grove’s Dictionary of Music and Musicians” has called Mendelssohn’s “Midsummer Night’s Dream” overture, Op. 21 “the greatest marvel of early maturity that the world has ever seen in music.” This work was completed by Mendelssohn on August 6, 1826 when Mendelssohn was 17 years and 6 months old.”—Source
For people known as GREATs’, 21 doesn’t seem to be very early.
Claim: [4] has been in preparation since [5].
Example: Mozart’s dad started him on a program of intensive training at the age of three.
Claim: Scientists created [6] for [5a]?
Example: It looks here, that precocity index is used much before the paper that is cited above, about the precocity index of musicians.
It seems like it was not created for comparing Mozart to his contemporaries.
Claim: [6] measures [7], “roughly”.
Example: Mozart has a precocity index of 130%, which is nothing but based on a “simple formula”:
-X/(Y-X)
X- Number of years of preparation before publicly playing a piece for average person Y- Number of years of preparation before publicly playing a piece for Mozart
Claim: [8] scored [9].
Example: All this probably requires is a citation?
Claim: [10] scored [10a]
Example: All this probably requires is a citation?
Claim: [11] is probably due to [12].
because
In Tiger’s case (his father)[13] never really claimed any (inborn talent)[14], but he thought that the (boy seemed to grasp things)[15] rather quickly. And (both of them)[1] state (Hard Work for the Success of Tiger)[2].
Claim: [13] never claimed [14].
Example: A quick Google search of “inborn talent tiger woods” does not come up with any news articles or media where [13] states [14].
Claim: [15] was rather quick.
I don’t know how I can find an example for that.
Claim: [1] state [2].
Example:
“People don’t understand that when I grew up, I was never the most talented. I was never the biggest. I was never the fastest. I certainly was never the strongest. The only thing I had was my work ethic, and that’s been what has gotten me this far.—Tiger Woods”
If you look at (Jack Welsh, CEO General Electric)[1], one of the (twentieth century’s manager of the century)[2] apparently showed no (inclination towards business until his mid-twenties)[3]. He started working in (chemical development operation at GE around that time)[3a]. And until that point there seems to be (nothing)[4] indicating the (business tycoon that he was going to become)[5]. Talent Waar ben jij?
Claim: [1] is [2].
Example:
“Jack Welch is a celebrated, legendary CEO. In his two decades at the helm of General Electric, he grew revenues to $130 billion from $25 billion and profit to $15 billion from $1.5 billion.”—Source
Claim: [1] showed no [3].
Example: By the age of 25 he seems to have finished his masters and PhD in Chemical engineering. He was even looking for jobs as a faculty in universities like West Virginia before he joined GE.
Claim: [1] was working in [3a].
Example: Its a question of fact. I guess I just cite a source. https://en.wikipedia.org/wiki/Jack_Welch
Claim: Until his mid-twenties, there was [4], indicating the [5].
Example:
By the age of 25 he seems to have finished his masters and PhD in Chemical engineering. He was even looking for jobs as a faculty in universities like West Virginia before he joined GE.
If talent existed and (refused to show itself even after so many years of life)[6], it beckons if (inate ability)[7] (talent) even exists.
Claim: If [7] exists and it [6], then [7] doesn’t exist
Example: unable to give examples for this if-then/proof-type statements
Maybe (talent)[8] seems like it doesn’t exist, but surely (intelligence)[9] and (memory power)[10] should have a high influence. Spoiler Alert! (Nope)[11].
Claim: [8] seems to not exist
Example: By the age of 25, Jack Welch, the ‘manager of the century’ didn’t even begin doing anything related to business and was considering working as a faculty in universities before he joined GE in Chemical Engineering.
Claim: [9] has high influence on Greatness/Success
Example: In a study of 45 thousand salesmen, whose IQ was pitted against their Sales ratings, it appears that intelligence showed a correlation of 0.04 with objective sales, whereas Achievement (Striving for competence in ones work) showed a correlation of 0.4 with objective sales.
So, Absolutely NOT!
Claim: [10] has high influence on Greatness/Success
Example:
“A study with highly skilled chess players and non-experts in chess was done where all were shown real chess game positions of 25 pieces for 5-10 seconds. The chess masters were able to recall the position of every single piece, whereas the non-experts were able to recall 4 or 5 pieces. As expected. This was followed up with random placement of chess pieces and the same 5-10 seconds to remember each piece. The chess masters and the non-experts pretty much ended up with the same results.”— from Agent18’s blog
So, Absolutely Not!
A study was conducted in the business realm. (Salesmen)[12] were an (attractive subject for this study)[13] as it is rather clear to measure (output/success)[14]. (More number of sales)[14a] implies (more success)[14b]. (The study)[15] was the largest of its kind containing (data of several dozen studies amounting to 45k individuals)[16]. Because of such a large number the (endless sources of noise)[17] are expected to be drowned. (The bosses)[18] gave (good indication of the IQ of the person with their ratings)[19], and with the help of (sales they actually made)[20], (the results)[21] were compiled.
Claim: [12] are [13].
Example: As a Design Engineer the contribution in terms of numbers ($ contributed to my company) is highly unclear. I guess as a result we have vague criteria for determining our impact such as “how I did my work in a year rated from 1-3” and “what I did rated from 1-3”. One day I work on a verification procedure, another day I work on some stage design which takes 2 years to make, whose value is not yet known. Where as in Sales, its OK/NOK. You either sold 5 bulbs or you didn’t.
Claim: [12] are [13] as it is clear to measure [14].
Example: You either sold 5 bulbs or you didn’t.
Claim: [14a] implies [14b]
Example: If you sold ‘n’ tables for X $. If you sold ‘2n’ tables then you make 2X $.
Claim: [15] was the largest of its kind
Example: There are other papers with sample sizes ranging from 11 to 16k. This study had almost 46k large sample. (It was actually a combination of several samples from different papers.)
Claim: [15] contained [16].
Example: [15] contained samples from studies with sample sizes from 11 to 16k.
Claim: With 45k samples, [17] is expected to be drowned
Example: This is a hard one, need to spend a lot of time on understanding randomness and come up with examples! Skip for now! I have no idea of examples for 17 in the context of the sales people, nor do I know why it is drowned or have an example for it.
Claim: [18] have [19].
Example: The bosses rated their staff on their performance and it turns out that they have 0.4 correlation with IQ. The bosses ratings were also correlated with Achievement but with 0.2 correlation. It looks like bosses have a better vision on IQ than anything else.
Claim: [20] was used as a outcome
Example: “Interest appears to be a strong predictor of sales (0.3 correlation)”.
(Intelligence)[22] was (virtually useless in predicting how well a salesperson would perform)[23]. Whatever it is that makes (a sales ace)[24], it seems to be something other than (brainpower)[25].
Claim: [22] was [23].
Example: 0.04 correlation between General Cognitive ability and objective sales
Claim: [25] is not useful for [24]
Example: 0.04 correlation between General Cognitive ability and objective sales
(Another investigation on real world performance)[1] was with (betting of horses)[2]. (The goal)[3] was to forecast (post-time odds)[4]. Based on (this)[5] the (classification of experts and non-experts)[6] was done. (Both groups)[7] seem to have (not much differences)[8] in terms of (experience at the track)[9], (years of formal education)[10], and (even the IQ averages and variation)[11]. Further investigation suggested that (IQ’s)[12] didn’t help (predict if someone was going to be good or bad at this)[13]. (A person with IQ of 85 (“dull normal”))[14] was able to (pick out the top horse in 10/10 races)[15]. And (a non-expert with IQ 118)[16] (picked up the top horse for 3/10 cases)[17]. There are a (dozen factors)[18] that go into deciding the (outcome of the game)[18], like (how the horse fared in the last game)[19], (track condition)[20] etc… Apparently the (low-IQ-experts)[21] used (far complex models that took a wide consideration of multiple variables)[22] unlike (the high-IQ-non-experts)[23].
To work this out, it looks like I need the original paper. But I can’t find a readable copy of it. So I skip this for now.
And this doesn’t stop here. (The same traits)[1] are observed with (Chess, GO and even scrabble)[2]. “Scrabble users show below average results on tests of verbal ability.”, And some Chess grand masters have IQ that are below Normal. All in all,
Claim: [1] is observed with [2].
Example: “Scrabble users show below average results on tests of verbal ability.”
(IQ)[2] seems to be a (decent predictor of performance)[3] on an (unfamiliar task)[4], but (once a person has been at it for a few years)[5], (IQ)[6] predicts (little or nothing about performance)[7].
Claim: [2] seems to be [3] on [4].
Example: I don’t have an example
Claim: [2] seems to not be [3] on [5].
Example: Chess grand masters have IQ that are below normal.
Claim: [2], predicts [7].
Example: Chess grand masters have IQ that are below normal.
But what about memory
The Czech master Richard Reti once played twenty-nine blindfolded games simultaneously. Miguel Najdorf, a Polish-Argentinean grand master, played forty-five blindfolded games simultaneously in Sao Paulo in 1947;
Surely (this)[1] is a (sign of the Divine)[2], right? Surprise, surprise! A study with highly skilled chess players and non-experts in chess was done where all were shown real chess game positions of 25 pieces for 5-10 seconds. The chess masters were able to recall the position of every single piece, whereas the non-experts were able to recall 4 or 5 pieces. As expected. This was followed up with random placement of chess pieces and the same 5-10 seconds to remember each piece. The chess masters and the non-experts pretty much ended up with the same results.
Claim: [1] is not [2].
Example: Despite Chess players seeming to have great memory (Richard Reti playing 29 blind-folded games), they still suck as bad as non-experts as they can only recall 4 or 5 pieces when the chess pieces are placed at random.
(The chess masters)[3] did not (have incredible memories)[4]. What they had was an (incredible ability to remember real chess positions)[5].
Claim: [3] did not have [4].
Example: When chess experts were asked to recall pieces placed in random on a chess board, they sucked as much as the non-experts.
Claim: [3] had [5].
Example:
“A study with highly skilled chess players and non-experts in chess was done where all were shown real chess game positions of 25 pieces for 5-10 seconds. The chess masters were able to recall the position of every single piece, whereas the non-experts were able to recall 4 or 5 pieces. As expected. This was followed up with random placement of chess pieces and the same 5-10 seconds to remember each piece. The chess masters and the non-experts pretty much ended up with the same results.”
(Experts remembered about 5-9 chunks of information at a time on the chess board)[6] that allowed them to (recall the positions of the pieces)[7]. The same was observed with GO and Gomuku even.
Claim: [6] allowed them to do [7].
Example: Experts could recall only 5-9 pieces when the chess coins were placed at random. But were able to recall the entire board for real chess positions.
(Many decades of research)[8] have shown that (average short-term memory)[9] holds (only about seven items)[10]. (The capacity of short-term memory)[11] doesn’t seem to vary much from person to person; virtually (everyone’s short-term memory)[12] falls in the range of (five to nine items)[13].
Claim: [8] has shown [9] holds [10].
Example: The main article cited 29k times was written in 1956 and it is still being cited to this day i.e., 7 decades.
Claim: [9] holds [10].
Example: People who do not have years of experience in music, are able to identify 7+-2 tones with a number corresponding to 1 tone.
Claim: [11] does not vary much from person to person
Example: experts and non experts in chess were able to identify 5-9 randomly placed pieces.
Claim: [12] falls in the range of [13].
Example: People who do not have years of experience in music, are able to identify 7+-2 tones with 1 number corresponding to 1 tone.
As reflected later in the book (TIO, Chap 6), (remembering 49 games at once)[14] is still a (ginormous feat)[15] (not possible with this short term memory)[16]. More on this later.
Up until now it might seem that (we)[17] are just unstoppable forces who can all become (legends)[18]. But certainly there are (limitations)[19]. There are (physical limitations to achievement)[20] such as Death and diseases, (limitations related to age)[21], (personal dimensions)[22] etc… It appears that other than (physical limitations)[23], there is not really (clearly understood or proven non-physical inate abilities inhibiting our potential to success)[24].
Issues
I would like it if you can give me feedback for these especially. This I picked from the above sections.
Hypothetical or from the past
There are (many issues)[1] we haven’t been able to look into yet, so we expect there are other (high-impact areas we haven’t listed)[3].
Claim: There could be other [3].
Question: Could there be other [3]?
In this case, I could give a hypothetical example or an example from the past. Can you comment on what would be the way to go?
Example A: Until a few years back 80khours thought that the best places to work on were “reducing near-term life risks aka reducing global health risks” but when they explored that there were global catastrophic risks that could kill the entire planet and future generations, they have now changed their stance on where people should be working considering the impact.
Example Hypothetical: If medical research into ‘how to slow aging’ seems
largely promising (90% chance of making it with 10b $
with a 100 people extra), in delivering a mechanism that doubles the
human life expectancy, it could be beneficial to work on it as it
could save 95% * 7b expected people lives/100 = 66m expected people
lives per person working on it
Because
For the (same reasons)[1] we think it’s an advantage to work on (neglected problems)[2], we also think it’s an advantage to take (neglected approaches to those problems)[3]. We discuss some of these approaches in this section.
Claim: Due to [1], it is good to work on [2].
because
Do we continue to skip because, due to etc…
Given A and B make example for the rest
Given our take on (the world’s most pressing problems)[1] and the (most pressing bottlenecks these issues face)[2], we think the following (five broad categories of career)[3] are a good place to (start generating ideas)[4] if (you have the flexibility to consider a new career path)[5].
Claim: Given [1] and [2], it appears that following [3] is a good place for [4].
Question: Is following [3], a good place for [4]?
I am not sure how to give an example “Given [1] and [2]”?
Is this “bad writing”, how to identify it and skip it
Given our take on (the world’s most pressing problems)[1] and the (most pressing bottlenecks these issues face)[2], we think the following (five broad categories of career)[3] are a good place to (start generating ideas)[4] if (you have the flexibility to consider a new career path)[5].
Claim: It appears that following [3], is a good place for [4].
Question: Is following [3], a good place for [4], if [5]?
What does 4, even mean? What is the point of it? Should I bother myself or skip these type of seemingly shit sentences. Who cares about generating ideas? What is the point here?
In general, Usually
(Many of the top problem areas we focus on)[1] are mainly (constrained by a need for additional research)[2], and we’ve argued that (research)[3] seems like a high-impact path)[4] in general.
Claim: [3] seems like [4] in general.
Example: Working in MIRI as a researcher could save 57k lives and has a better bang-for-the-buck as compared to Climate change (about 1000 times better).
I don’t know what general means here so I skip it!
Contrasting
You can also (support the work of other researchers)[1] in a (complementary role, such as a project manager, executive assistant, fundraiser or operations)[2]. We’ve argued (these roles)[3] are often neglected, and therefore especially high-impact. It’s often useful to have (graduate training in the relevant area)[4] before taking these roles.
Claim: It is good to [1] in [2].
Example: As discussed earlier, AI safety is really quite neglected with 100 people working on it with 10m $. Neil Bowerman from 80khours is trying to add people required to fill the “talent gaps”. If Neil is able to add 10 more people and even claim 1% of their total impact that would be 570 lives saved just for his work in a few years. Contrast that to a DS job which saves 400 people
I think it is important to contrast it with something otherwise it is hard for someone to understand if it is good or bad. Agree: to always contrast?
How useful
You can also (support the work of other researchers)[1] in a (complementary role, such as a project manager, executive assistant, fundraiser or operations)[2]. We’ve argued (these roles)[3] are often neglected, and therefore especially high-impact. It’s often useful to have (graduate training in the relevant area)[4] before taking these roles.
Claim: It is useful to have [4] before [3].
Split:
For [4] before [3]: Neil Bowerman has a PhD (equivalent) in Physics, where he worked on existential risks of extreme climate change with a focus on providing emission targets.
Not sure how “useful” [4] is before [3] or how to even go about answering it!
This seems to be exactly what happened with Rob Mather from AMF who claimed that his sales experience helped him.
Facts
(Mozart)[8] scored a (130 percent on the precocity index)[9] whereas (his current contemporaries)[10] scored (thirty to five-hundred percent)[10a].
Claim: [8] scored [9].
Example: All this probably requires is a citation? agree?
If then proof-type statements
If talent existed and (refused to show itself even after so many years of life)[6], it beckons if (inate ability)[7] (talent) even exists.
Claim: If [7] exists and it [6], then [7] doesn’t exist
Example: unable to give examples for this if-then/proof-type statements
(These advances)[5] could lead to (extremely positive developments, presenting solutions to now-intractable global problems)[6], but they also pose (severe risks)[7]. (Humanity’s superior intelligence)[8] is pretty much the sole reason that (it is the dominant species on the planet)[9]. If (machines surpass humans in intelligence)[10], then just as the fate of gorillas currently depends on the actions of humans, the (fate of humanity may come to depend more on the actions of machines than our own)[1].
Claim: if [10] then [1].
I have no idea how to answer this claim, how do I give an example that will inform “if A then B”.
Could lead to
(These advances)[5] could lead to (extremely positive developments, presenting solutions to now-intractable global problems)[6], but they also pose (severe risks)[7]. (Humanity’s superior intelligence)[8] is pretty much the sole reason that (it is the dominant species on the planet)[9]. If (machines surpass humans in intelligence)[10], then just as the fate of gorillas currently depends on the actions of humans, the (fate of humanity may come to depend more on the actions of machines than our own)[1].
Claim: [5]/[3]/[1] could lead to [6].
Example:
AlphaGo, identified superior ways of playing GO which were previously considered rubbish by humans for thousands of years. Computers seem like they can go beyond what humans can see with years and years of work, within just a year. Similarly, it could be possible to cure cancer and other diseases.
how to do I give an example for “could lead to”? I don’t think I have given one above!”
Stats
I am currently in India on vacation. Trying to clock 6 hrs per day.
Day 1 to 5: 6 hrs each (only DP)
Day 6: 6-7 hrs (DP correction and editing)
Total time: 36 hrs 200 phrases and ~ 110 claims
DP stats
-
Key ideas (74 phrases 42 claims 15 hrs)
-
AI (45 phrases ~21 claims 6 hrs)
-
TOI (80 phrases 54 claims 9 hrs)
DP Correction:
-
key ideas (0.5/min)
-
AI (1/min)
-
TOI (1.33/min)
P.S to self
Lot of procrastination especially on the last day. It’s 3 am and I still ain’t done, luckily the DP correction got over smooth and fast. Every day I sleep late and wake up later and start later. First day I started at 9 and within day 6 (today), I stared at 2. Something or the other came up.
Biggest source of distraction: Big ass tv with great movies. Movies shoudl be used only as a treat.