To Data Science or Not to Data Science
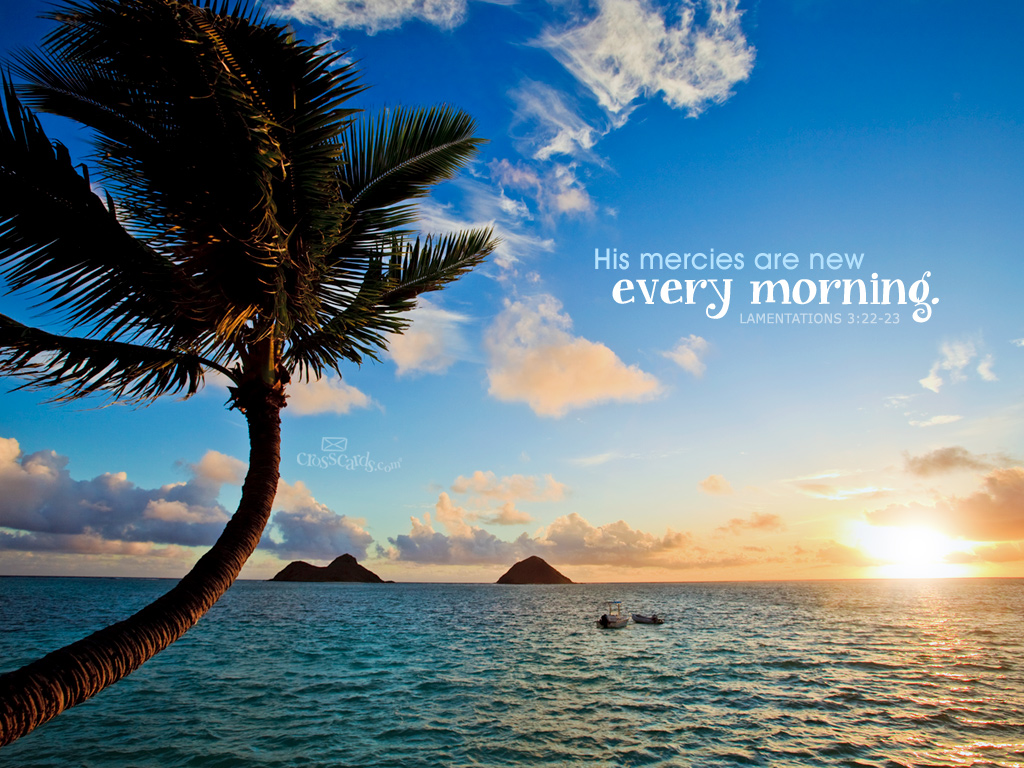
Entry Question
What should I do now? Careerwise.
Introduction
Last year, I made lifetime impact estimates for a career in Data Science and also a career as a GiveWell Researcher. The effective value estimates seem to suggest to bet on becoming a GiveWell Researcher.
Effective value | low | Best Guess | high |
---|---|---|---|
DS Masters | 713k | 741k | 865k |
DS L1 | 642k | 662k | 875k |
GW Researcher | 914k | 1.43m | 1.95k |
However, an STM seems to think I should pursue Data Science like a madman and give up the plans of becoming a GiveWell-type Researcher. His two main points are that, what I have are estimates (meaning that they are not “concrete dollar figures”). And secondly, gaining “EA skills” is far less tangible than “Data Science skills”1.
With this post I hope to explore these views and answer for myself what I should be doing in the coming months.
Estimates Inherently With A Lot Of Guesses
The effective value calculations shown above seem to suggest to bet on the GiveWell research path. But hello! What are the error margins?
The estimates computed for the “lifetime impact of a researcher” are essentially fermi-type estimates, which are based on a lot of guesses. For example, the counterfactual contribution of GiveWell is estimated to be 37%. This is obtained by assuming that a GiveWell-like organization would have formed anyway, after 7 years. If this number is 2 years instead of 7, then the estimate becomes 14%. If it is 10 years then the estimate becomes more. Changing the counterfactual contribution of GiveWell alone to 14% (from 37%), brings the total impact down to 800k from 1.4m.
The whole thing being based on a hypothetical scenario, makes it impossible to know what the real value would ever be. It is impossible to test this. And the sad part is that there are many such factors (e.g., counterfactual impact of a researcher, growth rate attributed to researcher etc.).
Typical fermi-estimates are often expected to be within a factor of
10. And in this case (lifetime impact of a researcher), I feel no
more confident than saying the estimate could be off by a
factor 10. This means a variation in the estimate could be between
143k to 14m$
. I don’t even know on which side of the error band the
impact could be in.
Things get even more blurry if it is not a GiveWell Research position–where the money moved is typically known. If it is something like Rethink Priorities–which mainly produces research–then you don’t have a clear dollar figure on how much money they influence. This means more guesses which you can’t verify or test.
However, I feel much more confident in the “estimates” on lifetime impact of a Data Science career. For one, they are not pulled out of thin air, they are actually based on historic data. For example, the probability of getting H1B within OPT years is estimated to be 58%. This is based on historic data on the number of people who make it through the lottery in one year (41.9%) and the number of people who make it out of an RFE (60.4%) 2.
In cases where such historic data is not available, anecdotal data is used to make a guess. For example, to estimate the probability of finishing master’s and getting a job in the US (in a “non-recession year”), I think of all my friends who are currently in the US and stories of people who had to come back as a result of not getting a job. Except for one guy who didn’t manage a proper job, all of the others (some 20 people) seem to have completed their masters and gotten a job in the US (even the supposedly “dumb” ones). Thus, I consider this probability of finishing Masters and getting a job in the US to be 70-80%.
The best part with choosing such a career is that I WILL KNOW what my contribution is going to be at the end of each year in Data Science, based on the donations. As a result it is way more tractable.
To sum up, I feel way more confident about the Data Science estimates than the estimates for a GiveWell Researcher position. I would like to however see other arguments in favor of one or the other.
Upskilling To Get A Foot In The Door
I recently wrote about EA skills needed based on a discussion with a couple of “EA people”. Skills listed include things like “ability to read academic papers”, “ability to write independent research”, “writing good conversation notes”, “quickly evaluating interventions” etc. In addition, looking at job postings show certain other skills like “cause area prioritization”, “gaining good calibration” and “funding opportunity investigation” etc.
Assuming I know what sort of skills are needed in the EA world, it appears to be hard to design a deliberate practice routine in some cases.
Some skills such as “reading academic papers”, I have hopes of practicing with a lot of feedback. For example, I can look at the papers cited in GiveWell, pass my own comments about the papers and then see what GiveWell had to say, compare and improve. There are a lot of intervention reports, so there is no dearth of material. I have elaborated on this routine here.
Similarly, for writing independent research (following on the Deliberate Practice case study here)–such as done by Saulius here–I have the hope of learning, by looking at blog posts and intervention reports, checking them against dimensions (specified in good reasoning transparency, “how we evaluate a study”, and some notes by Micheal Arid), and imitating them (#Ben-Franklin DP Method).
However, when I think of “cause area prioritization”, “funding opportunity investigation” or “gaining good calibration”, I am unsure how to proceed, other than by reading some documents from say Global Priorities Institute and “try to emulate” “their style”. This is very vague and I have low confidence of developing in it without feedback from an actual person.
I also think skills such as “conversation notes writing, based on audio”, “quickly evaluating interventions” or for that matter learning about “causal inference”, are going to be hard to upskill in, due to the lack of practice material (or feedback).
Contrast this to Data Science skills: Machine Learning, SQL, Python etc., which have tons of tutorials and courses to learn from, to the point of even getting a job in it. With a week of learning and completely using online resources, I recently got an internship offer, and from another company a “positive-ish response”3 based on assignments on web scraping and unsupervised classification respectively.
And even things like “exploratory data analyses” perhaps can be learned from the many problems in Kaggle and looking at the discussions. Not too straight forward but seems possible for practicing.
And in the worst case, I could do a masters or a post masters as suggested by several hiring managers from my company. Not sure what the stats on that are (I can find out) but they said they would “be interested” in me after that.
(Granted I wanted to port to a Data Science job, but now am “only an analyst” after like a year and that too only within my company. I really suspect it’s the “lack of experience” and the “lack of a degree”, as that is the feedback I seem to be getting from those willing to offer it. I am really not sure it is my “skill building in Data Science” which was the issue here. To become “great” however is a whole different story all-together.)
With EA I am really unsure what skills to focus on NOW. I recently got the feedback after an interview that I needed to think in a Bayesian way and focus on cost-effectiveness analysis, another feedback was that I needed to do independent research and practice forecasting and get credentials in it. And when I talk to other GiveWell-type researchers the feedback was to learn to read academic papers and write independent research. These seem like very different things needing a “lot of my time”. And to add to the confusion, I don’t really know how long or when the training is good enough, other than through applying or writing EA forum posts where people might weigh in on my level.
With Data Science also this part might be hard. People don’t seem to always be able to articulate what to work on and to what level to work on to get to a job. But my usual rule of thumb is to work on one example problem for a particular skills (i.e., one Kaggle problem) and seeing it through. This seems to be working “pretty well” (not with getting an interview but with doing “well”3 in interviews and case studies at least).
As a result, I am more confident about learning Data Science skills (and perhaps “mastering” them one day).
Earning To Give In The US
Going to the US is a crucial part of Earning-To-Give. Earning for the rest of my life in the Netherlands will probably get me to 300k-400k donations over my lifetime, while even if I make it to the US in another 20 years and manage a 500k salary with a 250k donation for just 3 years, I would make twice more than what I could do in the Netherlands over a lifetime. Thus it appears to be very important that I make it to the US, now or even eventually.
In order to maximize my chances I think I would need to start with a master’s and in case that tanks, I can always come back to Netherlands (or perhaps go to Canada4) and continue to keep trying via an L15 or pursue other ways to go to the US6. One thing very important in this whole process is to “practice Data Science skills” and try to become “great” (more on what to work on and how to work on it later).
It could so happen that a master’s would involve a lot of debt. If I
am in the US and have a job for 1-2 years I can perhaps reduce the
debt “significantly”. If I fail completely and don’t end up getting a
job in the US even for 1-2 years, then I will have a large debt (say
100k$
). But the chances of that happening are low I think (20%ish)
as most of the people I know have managed some job in the US after
their masters. H1B might be an issue, but getting a job so far doesn’t
seem to be.
EA is not going to be missing me
The EA sphere is super competitive. Below are the acceptance rates at several programs:
Year | Org | position | AR |
---|---|---|---|
2018 | OPP | GR | <5% |
2018 | EAF | GR & Operations | 3% |
2019 | CE | CE entrepreneurship program | 11.7% |
2020 | CE | CE Entrepreneurship program | <1% |
2019 | CE | Internship Mental Health | <2.5% |
2019 | FWI | Research analyst Welfare specialist | 2.4% |
As a result of the competitiveness they are going to continue “just as usual” with or without me. No one is going to be “missing me”. And perhaps I can leave the research to other people who are already good at it, and perhaps people who don’t have the possibility to Earn-To-Give in tech in US.
Possibility Of Getting Back To The EA
Perhaps in the off chance that EA somehow looks like a lucrative option for me in the future (depending on how EA progresses) maybe I can offer my skills as a Data Scientist. Currently there seem to be some companies using Data Analytics 7 in the EA community.
And in the worst case, I can always produce independent research, to “add more value”, while Earning-To-Give.
Value Drift
I always panic thinking about the future and how it’s going to be. I feel afraid that as a result of not being in the EA-sphere (i.e., talking EA 24x7 as would be in an EA job) I am going to loose all my motivation to grind every single day. I think of grinding day-in and day-out for the next 10 years and get worried. And to that person (me) I say, test what you can measure, and test something you can measure now.
Over the last 4 years, I can easily claim I would have been doing at least 2 hrs a day of work (growing by every year). Over the last 5 weeks I have been averaging 25 hrs a week of work. I am even doing 5 hrs on a weekday per week. And during all these years when I have been grinding, all I had was an STM mainly and the rest of the motivation was all through self or through meetups or forum posts etc. Now I don’t know about the future and I could be very wrong, but if the past is any indication of the future, I think it is going to be fine.
I am probably going to learn more statistics, more about rationality. I am going to be writing more and developing in these things with time. I am going to have a much better understanding of motivation. With all these powerful tools, I predict the future is going to be just fine with regard to value drift and feeling motivated to grind for EA “day-in and day-out”.
Perhaps if my concern is value drift or not feeling motivated enough, often I find that listening to a podcast or seeing other great people brings the persuasion back. Perhaps attending zoom events regularly might still be an option.
Once I am in California it should be much easier I guess to feel persuaded to be “great” in Data Science.
Persuasion is a bitch but I think we gots it whence we get our persuasion from other Data Science people and EA people, and avoid persuasion by staying away for the most part from “other people”.
Go Data Science!
Statistics
20 hrs
3200 words
Footnotes
-
The weakness of the EA researcher estimates is caused by the fact that each researcher is much farther removed from the impact than a donor. If it were about soliciting donations, an EA employee could talk about the incremental amount raised by the pledge drives that they ran. Whereas a researcher puts up research, which affects recommendations, which then affects where people donate. I don’t know of a way around this except to take the stats with a grain of salt (i.e., discount the estimated impact).
You mentioned in your earlier post that the “EA path” involved “building the skills through DP hopefully”. Given the twists and turns in the data science app-ing, how sure are you that you know which skills are needed for EA research? Also, as I’d mentioned earlier, the results of “EA practice” seem a lot less tangible than “data science practice”. You have a lot of data on the concrete skills data science jobs are looking for. You can confidently work on those and get feedback. Not sure if you have data or feedback like that for EA research.
-
- 41.9% chance of success after one H1B attempt for Master’s pool
- 100% chance of getting an RFE
- Success of RFE is 60.4%
- It is possible to have 3 attempts at the H1B Lottery.
- Total probability of success in one year =
41.9% x 100% x 60.4%=25.3%
- Total probability of success in 3 years =
1-(1-25.3%)^3
=58%
Based on appendix footnotes in this article. ↩
-
I interviewed to large company (80000 people) recently for a Data Science role where they told me after going quite far in the interview process that they were positive about my assignment and my attitude but that I was a junior. And that they expect more jobs here or in Sweden and that we should try again after the summer. And that they like me.” ↩ ↩2
-
I’m in Canada. I was offered ~220k USD in Seattle and ~210k CAD in Canada. Honestly Canadian salaries at top companies are not that much lower. It is mostly the exchange rate and taxes that screw you over here. If you can avoid living in Toronto or Vancouver cost of living is far lower than US tech hubs.
https://www.teamblind.com/post/Why-arent-tech-salaries-higher-in-other-countries-eZqFMfQU
30% cut from personal experience 180k CAD vs 215k USD. However other things are also cut like no sign on , lesser refreshers and so on
I’m 230K CAD TC as an Amazon SDEII in Canada. Pretty similar to US SDEIIs before currency conversion
https://www.teamblind.com/post/FAANG-salaries-in-US-vs-Canada-Y5hfUbFQ ↩
-
I know people from India who moved to the US in Investment banking, Computer science etc. All they needed was ↩
-
Chris Olah although he is a legend made it to the US without an undergrad degree with an alien with extraordinary ability, I am just saying there are examples who are great who made it to the US. https://80000hours.org/podcast/episodes/chris-olah-unconventional-career-path/ ↩
-
Faunalytics does empirical research that could make use of data analysis. Humane League Labs does data analysis, but they’ve had a very small team for a long time, so I wouldn’t count on them hiring. You could possibly get a grant from EA Funds or (ACE’s research fund, if they start it up again) to do independent data analysis. The alternative protein space might use data analysis for marketing or whatever. RP is doing data analysis for other animal orgs (I think THL and MFA). —MSJ in EA forum DMs